Pan-Genome Graphs Help Identify SNPs, INDELs and Structural Variants with Higher Accuracy
Improved methods for analyzing next-generation sequencing (NGS) data is mission critical given inherent flaws with the current human genome linear reference. Building on years of experience developing pan-genome references to eliminate reference bias, Seven Bridges GRAF is now broadly available to researchers on all Seven Bridges platforms.
The next-generation Seven Bridges GRAF product portfolio is a fast and accurate graph-based method that can construct and utilize genome graphs with the GRAF Germline Variant Detection Workflow consisting of GRAF Aligner and GRAF Variant Caller, both incorporating improved algorithms and updated pan-genome graph references since our first publication, Fast and accurate genomic analyses using genome graphs, Nature Genetics, 2019.
The GRAF Germline Variant Detection Workflow constitutes the first in a series of GRAF solutions being delivered to the community for a variety of targeted applications. With this release, we hope to facilitate the adoption of graph-based references and collaborate with the community to advance the technology while providing a better solution for NGS data analysis.
In order to highlight how GRAF compares to other state-of-the-art solutions, a series of benchmarks were performed utilizing the GRAF Germline Variant Detection Workflow which contains two pan-genome graph references compatible with GRCh37 and GRCH38 assemblies. These pan-genome references contain the genetic variation from multiple sources capturing the diverse genetic composition of underrepresented populations around the world.
The fundamental promise of using a graph reference is enhanced variant detection sensitivity by making use of previously identified genetic variants, both during alignment and variant calling. For this reason, the false negative rate in variant calling on the Syndip sample for each pipeline was measured. The figures show the number of variants missed by each pipeline for every thousand variants that need to be detected.
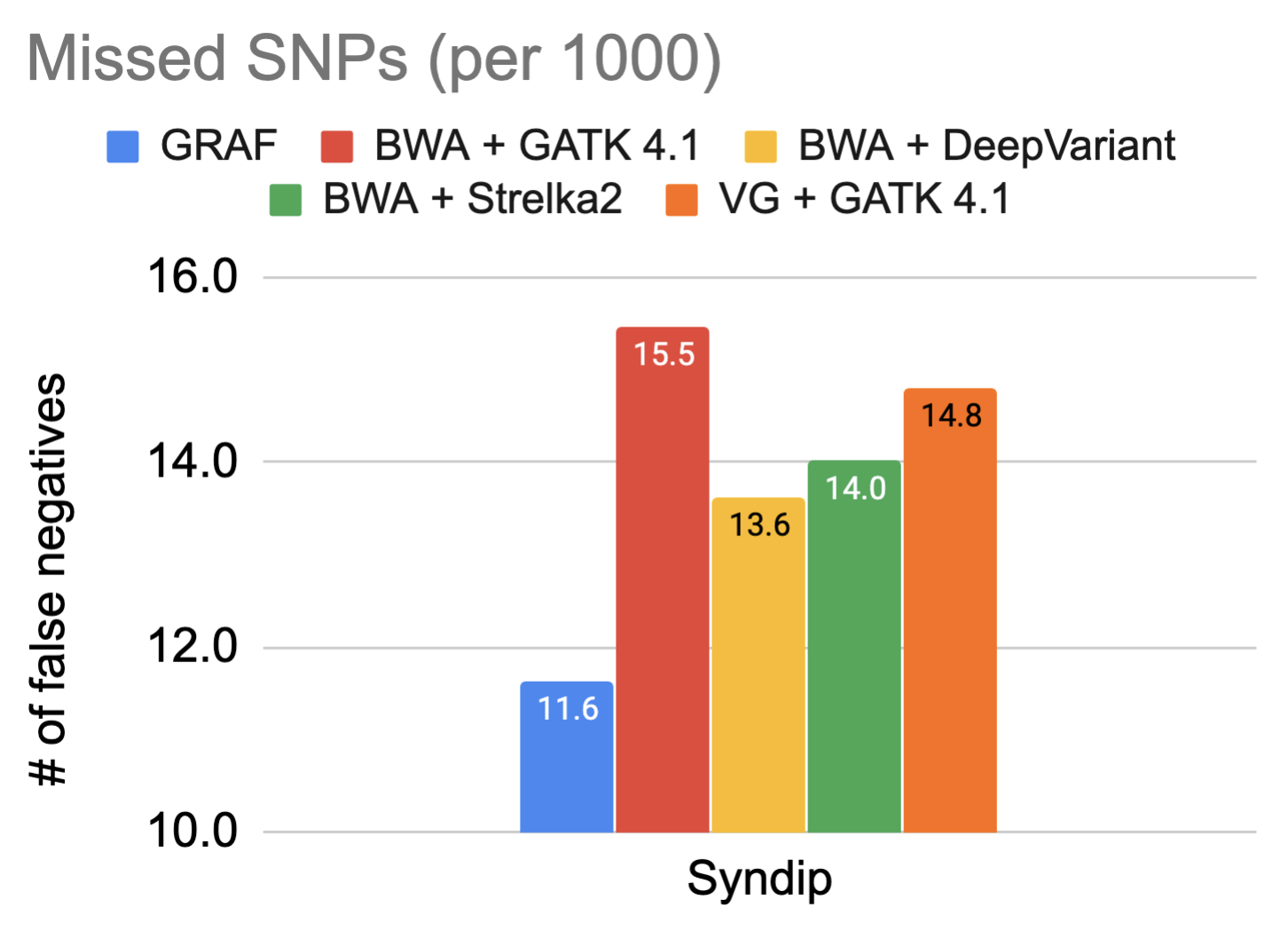
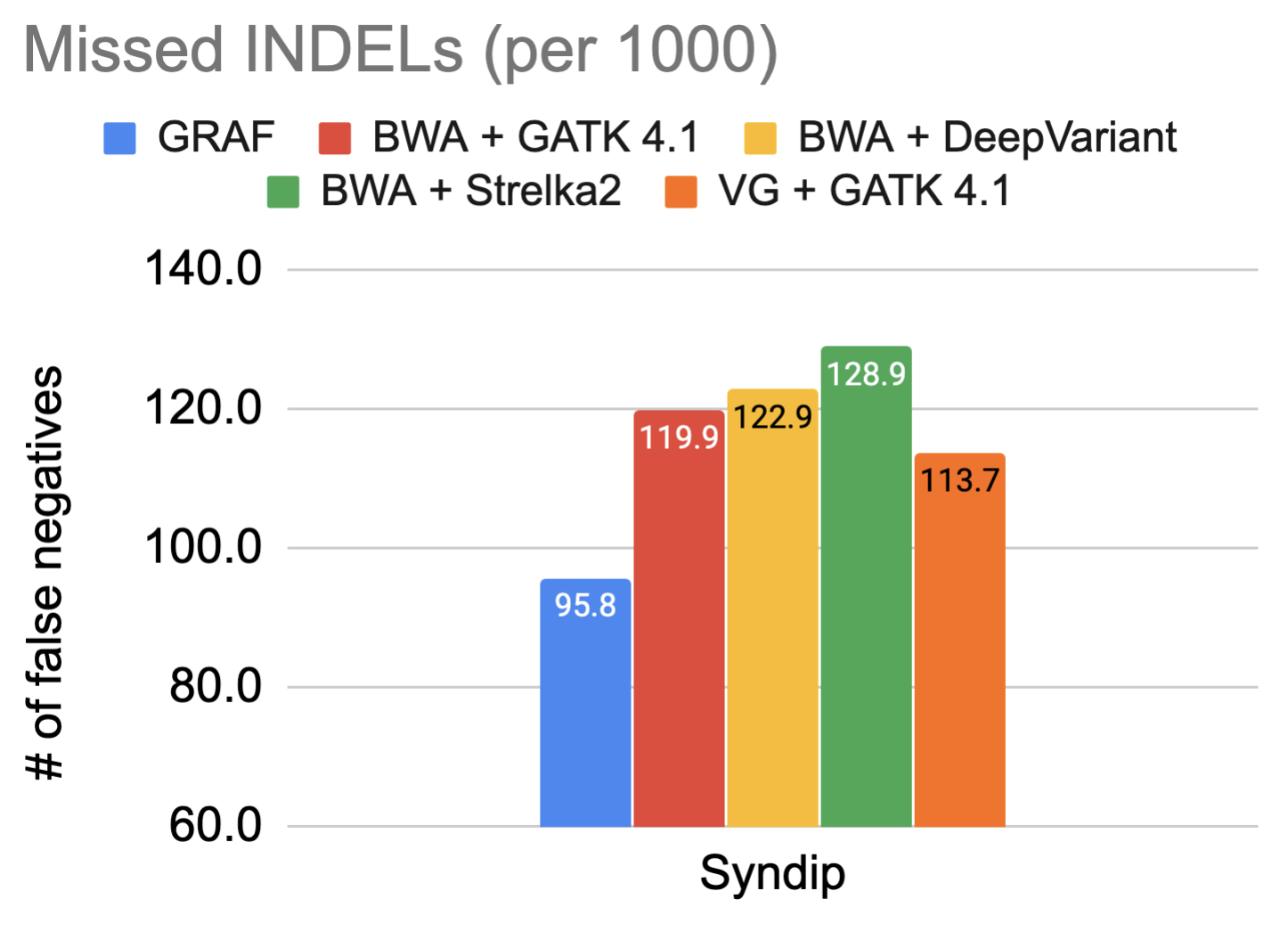
The data showcases that GRAF performs the best in both SNPs and INDELs. The false negative rate is much higher in INDELs compared to SNPs because INDELs are inherently more difficult to detect. This is exactly where we expect to see significant utility from graph references, so we dig one step further into these INDELs and plot the size distribution of true positive INDELs called by each pipeline below. GRAF has a significant advantage in sensitivity for long INDELs or SVs. This is an example of what can be achieved by using a Pan-Genome graph.
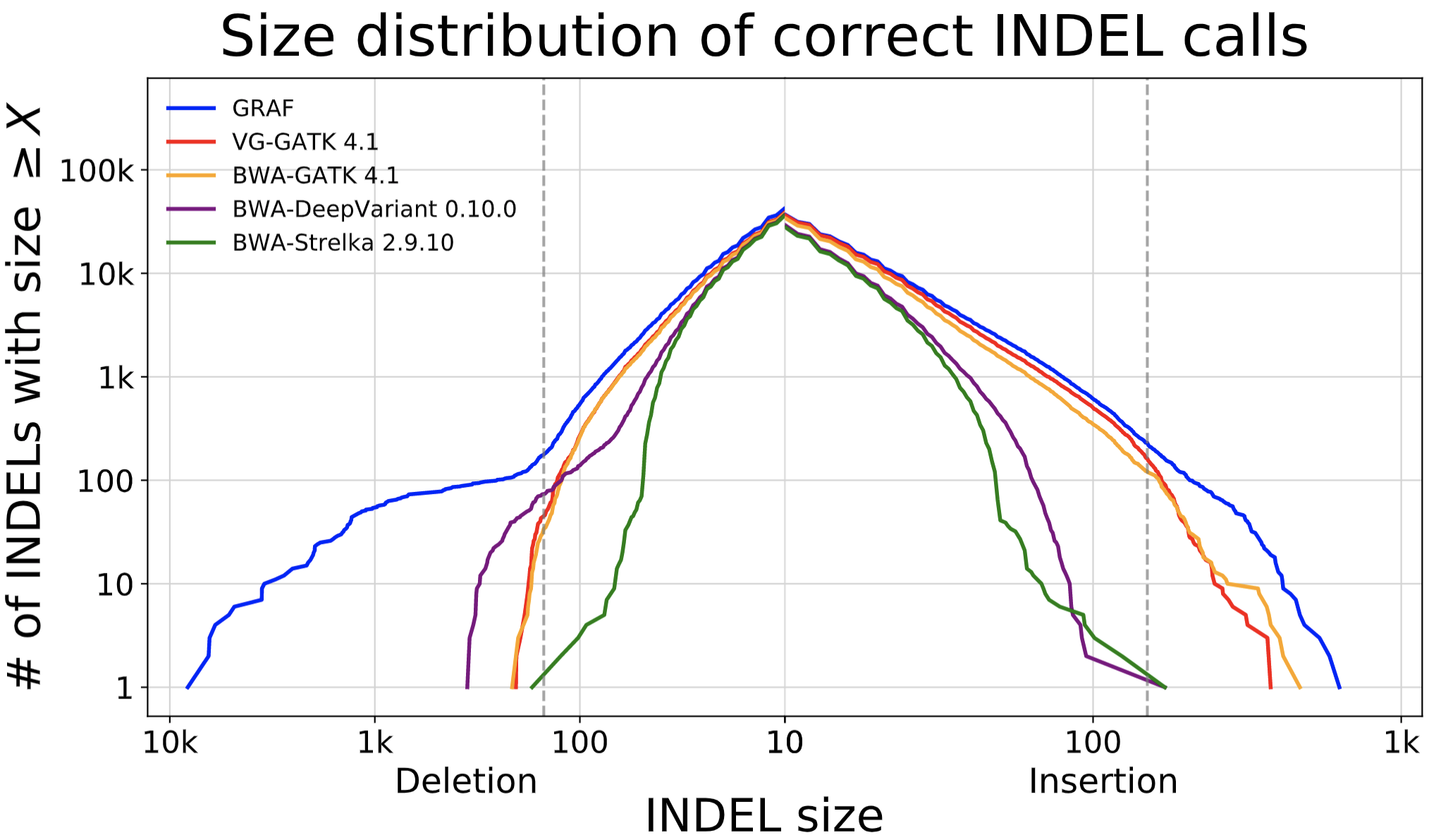
As an overall measure of accuracy, F-scores on the Syndip and the GIAB HG002 sample are provided and also showcase that GRAF has the highest SNP and INDEL f-score for the Syndip sample, which has a more comprehensive truth set compared to the GIAB samples. For HG002, GRAF takes first place in INDEL f-score while BWA + DeepVariant has the highest f-score for SNPs.
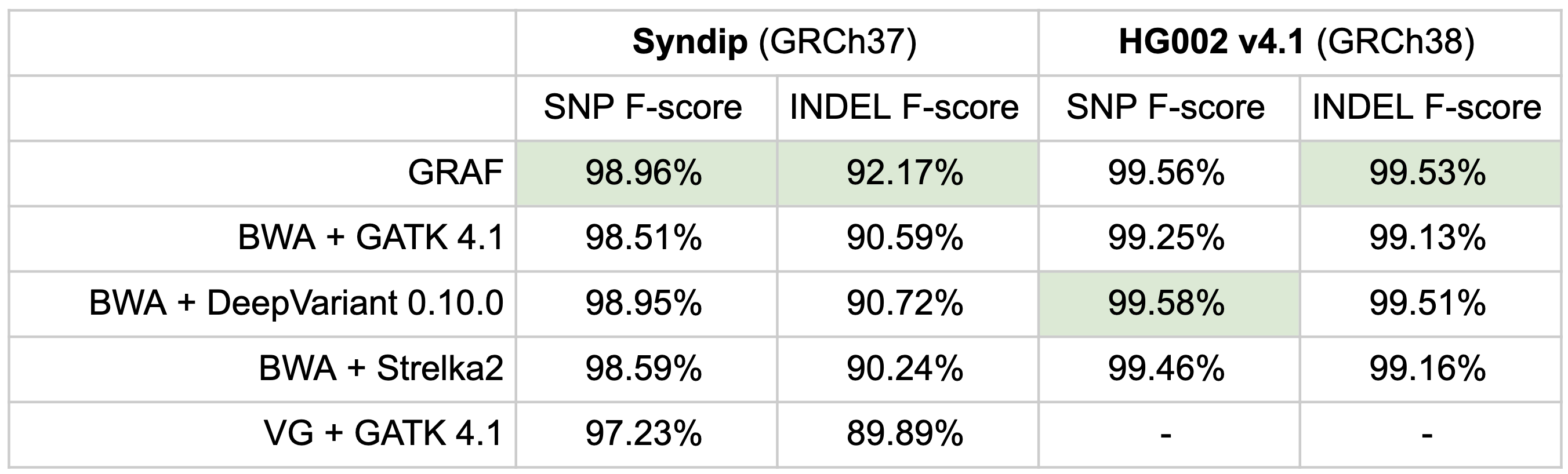
Finally, variant-calling precision of the pipeline on the same samples was compared. GRAF exhibits comparable precision to other pipelines, though not unambiguously superior. This marginal specificity impairment is acceptable in the context of clearly superior sensitivity, as highlighted by F-scores above. Also, we showed in Rakocevic et al that a few of the apparent false calls made by the GRAF pipeline in high-confidence regions are actually true variants, confirmed by Sanger sequencing of the samples. These variants are missing from the published GIAB truth sets, probably because the high-confidence ‘truth’ set was curated from the calls made by standard variant calling pipelines.
A top priority for GRAF product development is to ensure the algorithms are computationally efficient. With exponentially increasing amounts of genomic data, it becomes vital to make algorithms as efficient and cost-effective as possible to enable genomic analyses at the population level involving thousands of people, if not millions. Therefore, the average runtime (wall-clock time) from FASTQ to VCF for all pipelines on a collection of whole-genome sequencing samples with 30x coverage were measured. The data highlights that GRAF is fastest among the pipelines that have been tested so far, despite the extra information brought to the analyses by a graph reference.
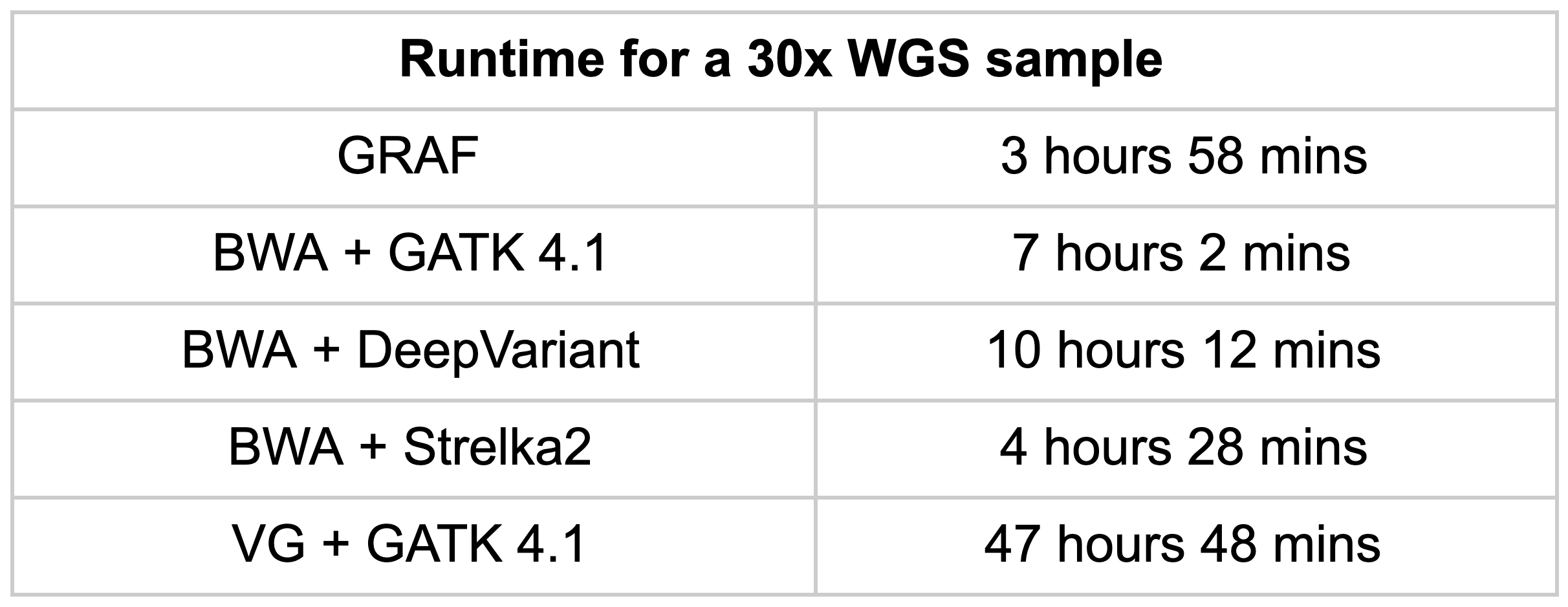
All of these results are, of course, just a hint of the extensive tests run on the Seven Bridges GRAF product portfolio.It is also noteworthy that GRAF uses the standard file and data formats for all analyses. Raw reads can be provided in FASTQ, CRAM or BAM files. GRAF Aligner reports alignments in either BAM or CRAM format, and GRAF Variant Caller produces a VCF file containing the variant calls, with GATK-compatible annotations. Even the genome graphs are stored in a VCF file so that it is easier to interact with using the vast amount of toolkits available for VCF manipulation.
Be sure to sign-up for our newsletter to receive future blog posts that will provide details on how to construct genome graph references and expansion of the GRAF portfolio for more targeted genomic applications.
GRAF is currently available for free on all Seven Bridges platforms. To learn how to get started, Contact Us today.