Exploring the Science Behind Neoantigen Discovery from Mutations to Proteins
Contributors: Julie Gil, Ph.D., Sarah Kleinstein, Ph.D., Cera Fisher, Ph.D., Dennis Dean Ph.D., and Kristina Clemens Ph.D.
During the COVID-19 pandemic, many of us gained first-hand experience of the efficacy of mRNA vaccines. Twenty years of dedicated research in the general application of mRNA vaccines fueled the rapid delivery of the specific and extremely effective inoculation for a virulent and incredibly awful new illness (here, your author speaks from personal experience). But vaccine usefulness doesn’t stop at your annual covid and flu shots. Vaccines teach your immune system to recognize foreign molecules on the surfaces of viruses, bacteria, or other cells–even those that started out as regular cells your body, as cancer cells do. To that end, several vaccines targeting specific cancers are currently undergoing clinical trials, building on the success of COVID-19 vaccine development trials.
As Table 1 from Dolgin et al.[i] shows, each of these cancer vaccines targets several neoantigens. As we discussed in our last blog post, neoantigens are molecules present on the surface of cancer cells that reflect mutations within the cell. Vaccines can activate the immune system to recognize and kill the cancer by delivering antigenic products via mRNA or DNA packets. But how do we identify those targetable neoantigens? One way is to brute-force test each small molecule and protein to be produced in a cancer cell to determine if it’s immunogenic. But that’s expensive and inefficient. A better way is to use computational tools to help whittle down the list of potential neoantigen candidates that these vaccines can effectively target. This week we want to dive into the steps of Velsera’s neoantigen workflow and explore best practices in the field that we and others have implemented to ensure efficient and accurate neoantigen discovery.
Table 1: Overview of current clinical trials targeting neoantigens. Table from Dolgin et al.
To briefly review, to most effectively prompt the immune system to target and kill cancer cells, vaccines need to mimic neoantigens that 1) are expressed in tumor but not normal tissue and 2) reflect the accurate protein sequence for the patient 3) are compatible with the patients HLA type so that the neoantigen will be expressed on the surface of cancer cells, and 4) be immunogenic; that is, prompt an immune response from the patient.
Combining Tumor-Normal DNA and Tumor RNA to improve Neoantigen detection
Myriad mutation types, several of which we discuss below, contribute to neoantigen generation. At the start of a new neoantigen discovery analysis, the user needs to determine how those mutations will be accounted for — via DNA sequencing (WGS/WES), RNA sequencing, or some mixture of the two. In cancer, pathogenicity is often driven by somatic mutations[ii]. Somatic mutations are mutations that arise in cells any time post-fertilization. These mutations can be leveraged to discover immunogenic neoantigens[iii].
The TESLA challenge (see our last post for more information!) was designed to be a collaborative competition to build best practices neoantigen discovery workflows[iv], and provided matched tumor-normal DNA and tumor RNAseq data to the teams to use in the development of those workflows. The TESLA challenge laid the groundwork for the development of neoantigen best-practices, but this is still an evolving field. Recent work supports a blended DNA and RNA sequencing approach to identify somatic variants likely to produce neoantigens [Nguyen[v],Hashimoto[vi]]. In particular, Hashimoto et al. found that a combined DNA and RNA approach (tumor-normal WES and RNA-seq) led to greater accuracy identifying somatic variants that were also highly expressed in cancer cells compared to tumor-normal RNA-seq alone.
Workflows solely using RNA have increased susceptibility to variant calling errors due to poor alignment around splice junctions, reverse transcription errors, and RNA editing sites[vii]. Furthermore, RNA-seq generally has lower coverage and potentially lower expression levels, which can cause additional variants to be missed[viii]. The Hashimoto paper notes that two large contributors to inaccurate predictions were the misclassification of germline variants as somatic, or outright missing somatic variants in regions of the genome with low coverage and low to no transcript count. As is increasingly being recognized across the scientific world, being able to leverage multiple types of ‘omics data, such as RNA and DNA, tends to greatly increase the likelihood of identifying actionable results.
Velsera recognizes that collecting both tumor-normal DNAseq data and tumor RNAseq data can be prohibitively expensive in terms of time and money to collect. The evidence, however, clearly shows that extra investments in sequencing yield better results overall. Money spent up front on robust data collection for in silico discovery workflows is an investment that pays dividends with more accurately predicted targets. This principle extends to the broader field of bioinformatics as well.
In a recent LinkedIn post, Brian Krueger, founder of BaseX Scientific Consulting, humorously argued that while longer RNAseq reads can be more expensive to generate, they are better able to pick up on RNA isoforms which may improve the diagnostic yield of the sequencing run. Brian’s points are as salient for neoantigen discovery as they are for bulk RNAseq. If RNAseq data is the only available input for neoantigen prediction, then users should ensure that their data uses longer read lengths at sufficient depth and quality to capture somatic variants and accurately measure the expression of candidate neoantigen sequences. Though as Brian points out, innovations like PacBio’s MAS-seq are helping to decrease sequencing costs by increasing read lengths while decreasing depth which may help to lessen the up-front cost of both DNA and RNAseq data collection for neoantigen discovery.
Selected elements of the Velsera Neoantigen Discovery Workflow
Haplotype phasing: which allele do you have?
For DNA, single nucleotide polymorphism (SNP) arrays and whole-genome / whole-exome sequencing (WGS/WES) methods have increased the volume of genotypic data available for analysis. However, without additional processing like haplotype phasing, it’s impossible to identify which alleles are co-located on the same chromosomes and which alleles were inherited from which parent of the patient. Haplotype phasing enables users to better identify the exact subset of variants the patient has in their genome and where they are located, meaning they can more accurately predict the resulting protein sequence of the neoantigen candidate. However, performing haplotype phasing can be a computational headache. Let’s just consider the human exome — regions of DNA that get transcribed into mRNA and subsequently translated into protein. The human exome is made up of 30 million base pairs, which is a massive search space for a single patient. When patient and sample sizes begin to scale, a global phasing approach can become prohibitively expensive in terms of both time and compute costs.
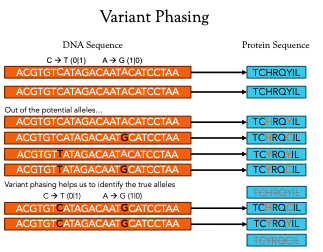
Velsera’s solution, which was developed in coordination with best practices identified as part of the TESLA competition [Wells et al], is to implement local phasing around known regions of variation. This results in windows of approximately +/- 30bp around the variant producing 10mer neoantigen candidates to assess for immunogenicity. This significantly cuts down the search space while providing critically important haplotype information within the most relevant regions of the genome. The smaller search space can decrease runtime and associated compute costs.
The role of mutations on neoantigen candidacy
As discussed last week, somatic mutations are a crucial component of neoantigen production within cancer cells. Velsera’s neoantigen workflow focuses on the impact of indels, proximal variants, and frameshifts caused by indels to help with the prediction of the protein sequence of neoantigen candidates around somatic variants. Each of these variant types influence the translation of the final protein product in different ways and ignoring any one of them can lead to the identification of a false neoantigen candidate. Figure 2 illustrates the effects of each of these variant types, but to really illustrate the effect of variants on neoantigen prediction, let’s focus on the simple case of a point (or single nucleotide) mutation.
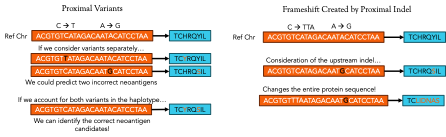
Figure 2: Different kinds of variants lead to different effects in the final protein product. Point mutations may just change a single amino acid while insertions and deletions can completely change the makeup of the neoantigen product.
Like any good, automated process, the system of codons that allows ribosomes to translate mRNA into proteins has built-in redundancies where different codons can lead to the same amino acid. Two common types of point mutations are silent or missense. A silent mutation occurs when a point mutation changes a single nucleotide in a codon that doesn’t ultimately change the final amino acid that the ribosome adds to the polypeptide. While these are important to keep track of, they will likely have little effect on the final neoantigen peptide sequence. A missense mutation, on the other hand, results in a different amino acid and could totally change the final configuration of the protein, meaning that any neoantigen mRNA or DNA sequence delivered in a vaccine that didn’t consider the mutation would be ineffective because it would be targeting an antigen with a completely different protein structure!
HLA-typing
Human leukocyte antigen (HLA) typing is the last step in the neoantigen selection process. HLA antigens fall into either class I, presented to CD8 + T Cells, or II, presented to CD4 + T cells[ix]. Identifying a patient’s HLA alleles and their corresponding Major Histocompatibility Complex (MHC) allows treatment developers to target neoantigens with high binding affinities for MHCs, which would increase the likelihood that those neoantigens would be present on the cell surface. A candidate neoantigen with a low binding affinity for MHC I or II may only be present in low volumes on the cell surface, if at all, and may not elicit a strong enough immune response to kill the tumor cells. Ensuring predicted high binding affinity of the candidate neoantigen increases the likelihood of that neoantigen being immunogenic.
Growing Towards the Future
Gene fusions are another important genetic mutation that can have a major impact on neoantigen prediction. Fusions occur when parts of different genes become fused together to make new gene sequences, usually through the process of chromosomal rearrangements in cancer cells[x]. They can disrupt a wide range of transcriptional regulation mechanisms, driving the development of tumor cells. Recent work by Weber et al.[xi], presents the utility of gene fusions for the detection of derived immunogenic neoantigens. This complex mutation event has produced immunogenic neoantigens on its own [Weber, Yang[xii]] and when combined with the host of alternative mutational events should serve to generate an ever-more precise list of candidate neoantigens. Recent publications even indicates that the tumor microbiota can give rise to neoantigens that prompt an anti-tumor response[xiii]. Velsera stays abreast of the advances in the field and will continue to iterate on our neoantigen workflow and best-practice approaches to ensure we are delivering the maximal value from our products.
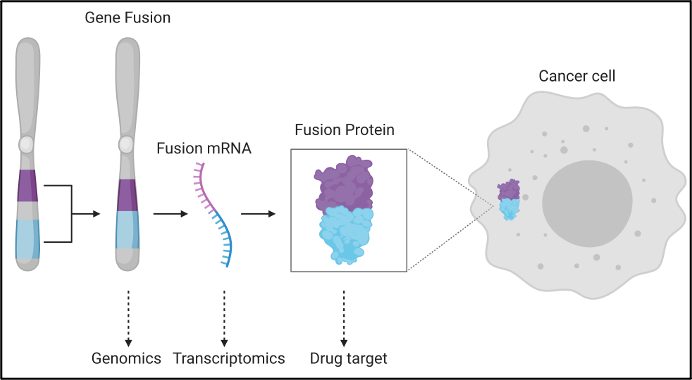
Next up
Bringing treatments to patients involves receiving FDA approval and requires regulatory reviewers to assess the implications of complex workflows like our neoantigen workflow. Neoantigen prediction workflows have the potential to add new complexities to regulatory reviews. For example, Individualized prediction workflows contain 100 tools or more with multiple connected functionalities. Interpreting workflows is also challenging due to the number of parameters, and often limited availability of standards for setting said tool parameters. Consequently, in our next blog, our Director of Translational Science, Dennis Dean, will discuss the importance of Bio-Compute Objects for FDA submissions and why the neoantigen workflow is a prime candidate for a regulatory submission use case.
Want to learn more?
Ready to accelerate your neoantigen research? Reach out to Velsera at hello@velsera.com.
[i] Dolgin E. (2023). Personalized cancer vaccines pass first major clinical test. Nature reviews. Drug discovery, 22(8), 607–609. https://doi.org/10.1038/d41573-023-00118-5
[ii] Martincorena, I., Raine, K. M., Gerstung, M., Dawson, K. J., Haase, K., Van Loo, P., Davies, H., Stratton, M. R., & Campbell, P. J. (2017). Universal Patterns of Selection in Cancer and Somatic Tissues. Cell, 171(5), 1029–1041.e21. https://doi.org/10.1016/j.cell.2017.09.042
[iii] Lang, F., Schrörs, B., Löwer, M., Türeci, Ö., & Sahin, U. (2022). Identification of neoantigens for individualized therapeutic cancer vaccines. Nature reviews. Drug discovery, 21(4), 261–282. https://doi.org/10.1038/s41573-021-00387-y
[iv] Wells, D. K., van Buuren, M. M., Dang, K. K., Hubbard-Lucey, V. M., Sheehan, K. C. F., Campbell, K. M., Lamb, A., Ward, J. P., Sidney, J., Blazquez, A. B., Rech, A. J., Zaretsky, J. M., Comin-Anduix, B., Ng, A. H. C., Chour, W., Yu, T. V., Rizvi, H., Chen, J. M., Manning, P., Steiner, G. M., … Defranoux, N. A. (2020). Key Parameters of Tumor Epitope Immunogenicity Revealed Through a Consortium Approach Improve Neoantigen Prediction. Cell, 183(3), 818–834.e13. https://doi.org/10.1016/j.cell.2020.09.015
[v] Nguyen, B. Q. T., Tran, T. P. D., Nguyen, H. T., Nguyen, T. N., Pham, T. M. Q., Nguyen, H. T. P., Tran, D. H., Nguyen, V., Tran, T. S., Pham, T. N., Le, M. T., Phan, M. D., Giang, H., Nguyen, H. N., & Tran, L. S. (2023). Improvement in neoantigen prediction via integration of RNA sequencing data for variant calling. Frontiers in immunology, 14, 1251603. https://doi.org/10.3389/fimmu.2023.1251603
[vi] Hashimoto, S., Noguchi, E., Bando, H., Miyadera, H., Morii, W., Nakamura, T., & Hara, H. (2021). Neoantigen prediction in human breast cancer using RNA sequencing data. Cancer science, 112(1), 465–475. https://doi.org/10.1111/cas.14720
[vii] Piskol, R., Ramaswami, G., & Li, J. B. (2013). Reliable identification of genomic variants from RNA-seq data. American journal of human genetics, 93(4), 641–651. https://doi.org/10.1016/j.ajhg.2013.08.008
[viii] O’Brien, T. D., Jia, P., Xia, J., Saxena, U., Jin, H., Vuong, H., Kim, P., Wang, Q., Aryee, M. J., Mino-Kenudson, M., Engelman, J. A., Le, L. P., Iafrate, A. J., Heist, R. S., Pao, W., & Zhao, Z. (2015). Inconsistency and features of single nucleotide variants detected in whole exome sequencing versus transcriptome sequencing: A case study in lung cancer. Methods (San Diego, Calif.), 83, 118–127. https://doi.org/10.1016/j.ymeth.2015.04.016
[ix] Ren, Y., Cherukuri, Y., Wickland, D. P., Sarangi, V., Tian, S., Carter, J. M., Mansfield, A. S., Block, M. S., Sherman, M. E., Knutson, K. L., Lin, Y., & Asmann, Y. W. (2020). HLA class-I and class-II restricted neoantigen loads predict overall survival in breast cancer. Oncoimmunology, 9(1), 1744947. https://doi.org/10.1080/2162402X.2020.1744947
[x] Glenfield, C., & Innan, H. (2021). Gene Duplication and Gene Fusion Are Important Drivers of Tumourigenesis during Cancer Evolution. Genes, 12(9), 1376. https://doi.org/10.3390/genes12091376
[xi] Weber, D., Ibn-Salem, J., Sorn, P., Suchan, M., Holtsträter, C., Lahrmann, U., Vogler, I., Schmoldt, K., Lang, F., Schrörs, B., Löwer, M., & Sahin, U. (2022). Accurate detection of tumor-specific gene fusions reveals strongly immunogenic personal neo-antigens. Nature biotechnology, 40(8), 1276–1284. https://doi.org/10.1038/s41587-022-01247-9
[xii] Yang, W., Lee, K. W., Srivastava, R. M., Kuo, F., Krishna, C., Chowell, D., Makarov, V., Hoen, D., Dalin, M. G., Wexler, L., Ghossein, R., Katabi, N., Nadeem, Z., Cohen, M. A., Tian, S. K., Robine, N., Arora, K., Geiger, H., Agius, P., Bouvier, N., … Morris, L. G. T. (2019). Immunogenic neoantigens derived from gene fusions stimulate T cell responses. Nature medicine, 25(5), 767–775. https://doi.org/10.1038/s41591-019-0434-2
[xiii] Wang, M., Rousseau, B., Qiu, K., Huang, G., Zhang, Y., Su, H., Le Bihan-Benjamin, C., Khati, I., Artz, O., Foote, M. B., Cheng, Y. Y., Lee, K. H., Miao, M. Z., Sun, Y., Bousquet, P. J., Hilmi, M., Dumas, E., Hamy, A. S., Reyal, F., Lin, L., … Huang, L. (2023). Killing tumor-associated bacteria with a liposomal antibiotic generates neoantigens that induce anti-tumor immune responses. Nature biotechnology, 10.1038/s41587-023-01957-8. Advance online publication. https://doi.org/10.1038/s41587-023-01957-8